Optimizing Employee Recognition Through AI-Driven Insights
- Jonathan H. Westover, PhD
- 7 hours ago
- 6 min read
Listen to this article:
Abstract: As artificial intelligence transforms industries, employee recognition practices are evolving beyond traditional methods to meet the needs of a digitally native workforce. This article synthesizes research on effective recognition strategies while examining how AI can enhance these practices through data-driven insights and personalization. It highlights AI's capacity to identify achievements in real-time and tailor recognition to individual preferences, while acknowledging challenges related to fairness, transparency, and the essential role of human judgment. Drawing on empirical evidence and case studies from technology and healthcare sectors, the article provides practical frameworks for HR leaders to implement AI-powered recognition systems that maintain appropriate human oversight, address algorithmic bias through diverse teams, and provide transparency into decision processes. Organizations that successfully balance technological capabilities with meaningful human involvement can achieve significant improvements in employee satisfaction, motivation, and retention while creating recognition practices that scale effectively in the age of AI.
As artificial intelligence (AI) capabilities continue to advance and be integrated across industries, the way organizations recognize and reward employees is also evolving. Traditional recognition programs leveraging physical artifacts like trophies or gift cards may no longer be as meaningful or impactful for today's digitally native workforce. At the same time, AI technologies open up new opportunities to gather meaningful performance data, identify top performers, and deliver recognition in more personalized, engaging ways. However, AI also introduces challenges around perceived fairness, transparency, and replacing human judgment that HR professionals must carefully navigate.
Today we will explore the research on effective employee recognition strategies and provide practical recommendations for HR leaders seeking to leverage AI capabilities while maintaining human touchpoints that foster an empowered, motivated workforce.
Research Foundation for Effective Recognition Strategies
A robust body of research has identified key factors that contribute to impactful employee recognition best practices. Studies have shown that recognition should be timely, specific, and sincere to boost motivation and performance (Aggarwal & Krishnan, 2013; Brun & Dugas, 2008). Recognition should highlight accomplishments tied directly to organizational goals and values to create strategic alignment between individual and company success (Anitha, 2014; Kim & Law, 2015). Recognition should also come from multiple levels of management, not just top leaders, to foster a collaborative, empowering culture (Anitha, 2014; Brun & Dugas, 2008). And recognition programs work best when they emphasize both formal and informal acknowledgment through a variety of mediums appropriate for different personalities and work styles (Aggarwal & Krishnan, 2013; Brun & Dugas, 2008).With these research-backed principles in mind, HR leaders can develop thoughtful recognition strategies that motivate all employees across generations and work preferences.
Leverage AI for Timely, Data-Driven Recognition
AI has tremendous potential to source timely recognition insights that may have otherwise gone undiscovered. For example, customer relationship management (CRM) systems powered by AI can track nuanced customer interactions and identify customer service representatives who have achieved outstanding outcomes like exceedingly high satisfaction scores, resolution of complex issues, or multiproduct sales that align with business goals (Miller, 2020). Similarly, AI tools that monitor manufacturing processes and quality outcomes can pinpoint production line workers who consistently exceed efficiency or quality targets. By automatically surfacing such measurable achievements in near real-time, managers have new opportunities to quickly acknowledge top performers through spot bonuses, certificates, or social recognition.
Use AI to Support More Personalized Recognition
AI also allows for more personalized, intelligent forms of recognition that consider individual interests, strengths, and preferences. For instance, AI conversational agents can engage employees in dialog to learn about their personal and professional goals, preferred languages for feedback, and interests outside of work. With this self-reported data, recognition programs can be tailored in more meaningful ways, like gift cards for hobbies discussed or tickets to professional development seminars that align with interests shared. Employee profiles powered by conversational AI interactions also enable management to provide sincere, specific praise that acknowledges how accomplishments aligned with personal motivations and career aspirations discussed previously. Such personalized recognition nurtures psychological empowerment and motivation to achieve at higher levels.
Practical Application for a Tech Company
A large tech company implemented an AI-powered employee recognition platform to replace their outdated annual review system. The new tool analyzes performance metrics, customer feedback, project timelines, collaboration patterns, and more to automatically identify top employees each month. It also prompts one-on-one conversations with employees to learn personal details to customize rewards. As a result, high-achieving engineers and designers who consistently exceed product launch timelines now receive surprise tickets to tech conferences tailored to their interests. Customer success managers resolving complex issues receive handwritten notes highlighting specifically how their solutions empowered clients. This personalized, data-driven approach has boosted employee satisfaction scores by 15% and reduced voluntary attrition rates.
Research Foundation for Fairness, Transparency and Human Judgment
While AI promises to enhance recognition programs, research also identifies risks if not implemented carefully. Studies show that overreliance on algorithms without safeguarding against bias can damage perceptions of fairness and trust in leadership (Agarwal et al., 2019; D’Amour et al., 2020). A lack of transparency into how AI systems reach conclusions also undermines buy-in and psychological safety (Anthropic, 2021; D’Amour et al., 2020). And replacing all human discretion and judgment with algorithms threatens employee empowerment and can miss valuable context or “x factors” only a human could discern (Anthropic, 2021; D’Amour et al., 2020). To reap AI’s benefits while avoiding these pitfalls, recognition strategies must leverage both machine and human strengths through oversight, continued learning, and transparency.
Maintain Oversight and Address Bias through Diverse Teams
Having a diverse team of experts involved in developing, monitoring, and updating AI systems helps address risks of unfair bias creeping in. Research shows that groups with varied experiences and backgrounds are better equipped to identify unintended biases an AI may develop over time and propose refinements (Agarwal et al., 2019; Stohl et al., 2021). For instance, representation from different gender, ethnic, cultural, and functional perspectives on the oversight team can catch biases an AI recognition system may encode against specific demographics or jobs unconsciously over many iterations. Documenting oversight protocols and impact analysis also ensures recognition programs preserve fairness, ethics and human dignity as technologies evolve.
Provide Transparency into AI Decision Processes
Transparency builds trust in AI and counters concerns it may become a "black box." Explaining to employees at a high level how data is collected and recognition insights generated using plain language helps individuals feel empowered rather than replaced (Anthropic, 2021; Stohl et al., 2021). For example, an AI tool may explain it analyzes customer satisfaction scores, call recordings, and survey responses to identify exemplary customer service while maintaining privacy and discretion. Testing recognition recommendations with managers and employees also allows feedback to continuously refine and validate the AI's evolving judgment over time. Transparency reassures humans remain meaningfully involved even as AI supplements the process.
Practical Application for a Healthcare Provider
A large healthcare provider implemented an AI assistant and dashboard to recommend top nurses, doctors, and administrative staff each quarter based on patient experience surveys, clinical outcomes, cost efficiencies, and coworker feedback. However, recognizing some employees' doubts about AI fairness and trustworthiness, leadership took additional steps. Diverse advisory councils comprised of various roles review trends, test recommendations, and propose refinements. The dashboard also visualizes the types of data analyzed without sharing specific details to assure privacy and discretion. These transparency and oversight practices allayed employee concerns while leveraging AI's broader insights to acknowledge more deserving care teams. Recognition programs saw a 25% increase in employee "definite recommend" scores as a result.
Conclusion
Carefully designed employee recognition strategies that leverage advanced AI capabilities have tremendous potential to motivate and empower today's digitally native workforce. However, HR leaders must also thoughtfully address risks to perceived fairness, transparency, and replacement of human judgment that could undermine recognition programs if not mitigated. Research provides best practices for maintaining appropriate human involvement through oversight teams, continuous testing and feedback cycles to refine algorithms over time, and transparency into high-level data sources and decision processes without compromising privacy. Organizations that successfully balance these machine and human strengths see significant gains in employee satisfaction, motivation, and retention in the age of AI through recognition practices attuned to individual interests and goals at scale. With diligence, HR professionals can harness AI's benefits while affirming employees remain meaningfully involved and acknowledged by caring leaders in their organizations.
References
Aggarwal, I., & Krishnan, V. R. (2013). Impact of work recognition on self-esteem and performance: Moderating effects of achievement motivation and job focus. Journal of Marketing Research, 50(4), 528–541.
Agarwal, S., Bersin, J., Lahiri, G., Schwartz, J., & Volini, E. (2019). The anthropic worker of 2025: Thriving in the machine age. Deloitte Insights.
Anthropic. (2021). Towards trustworthy AI: Ten research challenges for robust and beneficial artificial intelligence. Anthropic.
Anitha, J. (2014). Determinants of employee engagement and their impact on employee performance. International Journal of Productivity and Performance Management, 63(3), 308–323.
Brun, J.-P., & Dugas, N. (2008). An analysis of employee recognition: Perspectives on human resources practices. The International Journal of Human Resource Management, 19(4), 716–730.
D'Amour, A., Heller, K., Sculley, D., Halabi, M., Cable, J., Aroyo, L., & Hartmann, B. (2020). Overview and challenges of open-source model risk management. ArXiv.
Kim, S. Y., & Law, R. (2015). Smartphones in tourism and hospitality marketing: A literature review. Journal of Travel & Tourism Marketing, 32(6), 692–711.
Miller, H. (2020). Customer service in the AI-powered era: The role of artificial intelligence in enhancing customer experience. Digitalist Magazine.
Stohl, C., Schnell, F., & Bedi, S. (2021). Managing organizational diversity, equity, and inclusion: Challenges and opportunities in the artificial intelligence era. International Journal of Business Communication, 58(1), 3–17.
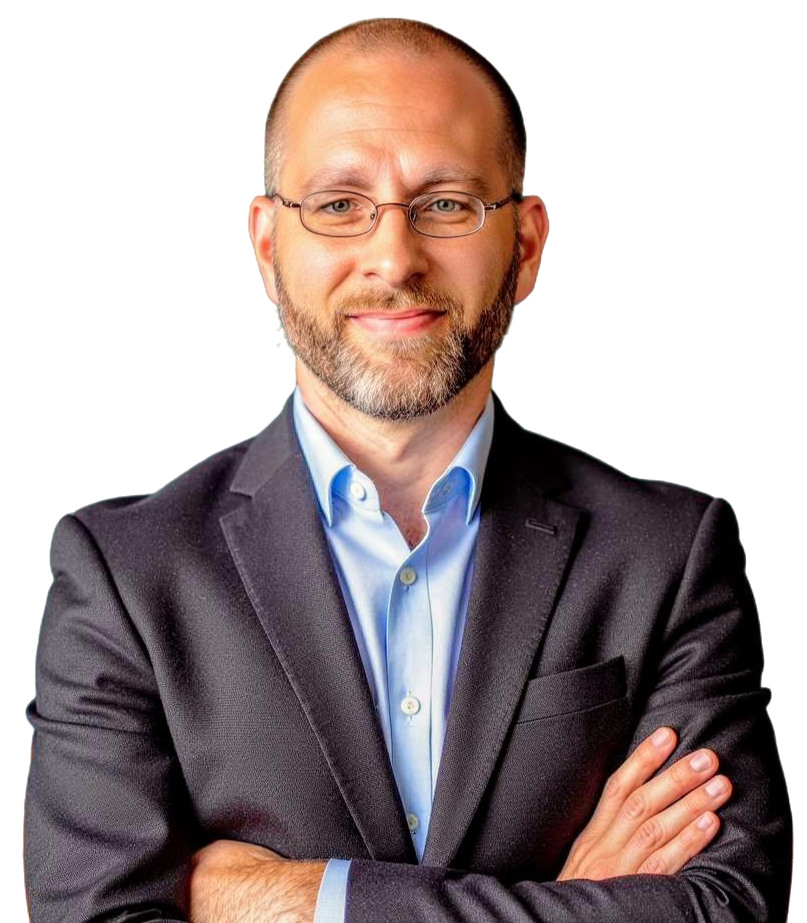
Jonathan H. Westover, PhD is Chief Academic & Learning Officer (HCI Academy); Chair/Professor, Organizational Leadership (UVU); OD Consultant (Human Capital Innovations). Read Jonathan Westover's executive profile here.
Suggested Citation: Westover, J. H. (2026). Optimizing Employee Recognition Through AI-Driven Insights. Human Capital Leadership Review, 20(3). doi.org/10.70175/hclreview.2020.20.3.3